To find clinically meaningful preoperative predictors of diabetes remission and conversely inadequate glycemic control after gastric bypass surgery. Predicting the improvement in glycemic control in those with type 2 diabetes after bariatric surgery may help in patient selection.
Preoperative details of 154 ethnic Chinese subjects with type 2 diabetes were examined for their influence on glycemic outcomes at 1 year after gastric bypass. Remission was defined as HbA1c ≤6%. Analysis involved binary logistic regression to identify predictors and provide regression equations and receiver operating characteristic curves to determine clinically useful cutoff values.
Remission was achieved in 107 subjects (69.5%) at 12 months. Diabetes duration <4 years, body mass >35 kg/m2, and fasting C-peptide concentration >2.9 ng/mL provided three independent preoperative predictors and three clinically useful cutoffs. The regression equation classification plot derived from continuous data correctly assigned 84% of participants. A combination of two or three of these predictors allows a sensitivity of 82% and specificity of 87% for remission. Duration of diabetes (with different cutoff points) and C-peptide also predicted those cases in which HbA1c ≤7% was not attained. Percentage weight loss after surgery was also predictive of remission and of less satisfactory outcomes.
The glycemic response to gastric bypass is related to BMI, duration of diabetes, fasting C-peptide (influenced by insulin resistance and residual β-cell function), and weight loss. These data support and refine previous findings in non-Asian populations. Specific ethnic and procedural regression equations and cutoff points may vary.
Surgery is the most effective treatment for clinically severe obesity. Although most patients display a dramatic improvement in type 2 diabetes, not every patient has remission of diabetes after surgery, with some not seeing substantial improvement and many having recurrence at a later date, sometimes in association with weight regain (1–5). Bariatric surgery, now often referred to as “metabolic surgery,” has been recommended as an effective treatment option for type 2 diabetes for those obese patients who do not have satisfactory control with lifestyle change (1). Optimal outcomes of surgical treatment of diabetes will be obtained if the patients best suited to the surgery are selected, but a clinically relevant grading system to categorize and predict outcomes of metabolic surgery is lacking (1). To develop such a grading system, the responses of patients to metabolic surgery need to be carefully observed, and the characteristics of responders that may have predicted their successful outcomes must be identified. Perhaps even more important is the identification of a group that does not respond well to metabolic surgery, so that such patients are not exposed to the unnecessary risk of surgery without expectation of clear benefit.
Many preoperative patient factors have been associated with outcomes, including age, diabetes duration, glycemic control (HbA1c), fasting C-peptide concentration, BMI, ethnicity, and medications used to manage blood glucose, including oral hypoglycemic agents and insulin (6–10). There are a number of weaknesses in many of these studies, and these include insufficient numbers to identify all independent clinically relevant factors, dealing in a univariate manner with a number of factors that are clearly related to one another, use of varying criteria for remission, and failure to record potentially important clinical predictors.
The primary aim of this study was to seek, in a clinically meaningful way, the preoperative predictors of diabetes remission (HbA1c ≤6.0%) at 12 months after gastric bypass surgery in a cohort of ethnic Chinese patients with type 2 diabetes who reside in Taiwan. Secondary aims included the identification of preoperative predictors of HbA1c ≤7.0% at 12 months, a comparison of the effectiveness of mini gastric bypass (MGB) and Roux-en-Y gastric bypass (RYGB) for weight loss and glycemic control, and examination of the relevance of weight loss after gastric bypass as a predictor of diabetes remission.
RESEARCH DESIGN AND METHODS
This is an analysis of data from a longitudinal study of the clinical predictors for the successful treatment of type 2 diabetes after metabolic surgery, as part of protocol US NCT0131797 9. Ethics approval was provided by the Min-Sheng General Hospital, Taiwan, and all participants signed informed consent to the study participation and surgery. Some of the broader outcome data of a subsection of this cohort have been published as part of a multinational collaborative study (8,9).
The cohort comprising the data set were diabetic patients with suboptimally controlled type 2 diabetes (HbA1c >7%). The patients were all required to have an acceptable operative risk to be considered for surgery and were aged 18–67 years. The exclusion criteria were the presence of end-organ damage, pregnancy, and previous gastrointestinal surgery. Participants were also excluded if the C-peptide was below 0.9 ng/mL.
Gastric bypass
Two methods of gastric bypass were performed. The first was standard RYGB with a 100-cm biliopancreatic limb and a 100- to 150-cm alimentary limb. The second was a simplified procedure, the MGB or “single anastomosis gastric bypass.” This procedure had been adapted and previously described (11). To describe briefly, with a standard 5-port laparoscopic technique, a long-sleeved gastric tube approximately 2.0 cm wide was created with the EndoGIA stapler (Tyco; United States Surgical Corporation, Norwalk, CT) along the lesser curvature from the antrum to the angle of His and divided from the remnant stomach. A Billroth II loop gastroenterostomy was created with the small bowel about 150 to 200 cm distal to the ligament of Treitz (biliopancreatic limb) with the Endo-GIA stapler. No drain tube was left. Patients were free to choose either procedure after being informed in detail about both.
Data extraction
HbA1c at 12 months after surgery was the primary outcome variable of interest. The preoperative variables listed were extracted from the database, in line with our aims and inferred hypotheses. They were then grouped in clinically relevant blocks to build the hierarchical logistic regression model. Block 1 consisted of age, sex, BMI, waist circumference, and waist-to-hip ratio. Block 2 consisted of duration of diabetes, family history of diabetes, use of oral hypoglycemic agents, and use of insulin. Block 3 consisted of HbA1c, C-peptide, homeostatic model assessment insulin resistance (HOMA) (12), HDL cholesterol, triglycerides, highly sensitive C-reactive protein (hsCRP), aspartate aminotransferase (AST), and uric acid. We also extracted the type of gastric bypass performed (block 4), and percentage weight loss at 12 months after surgery (block 5).
Baseline biochemical measures were assessed in the fasting state immediately before surgery, and assays were performed in a single accredited laboratory. The laboratory C-peptide reference range was 0.9–4.0 ng/mL. Postsurgical diabetes care management was performed by one of the hospital endocrinologists (L.-M.C. or K.C.) and the diabetes educator (S.-C.C.).
Analysis and statistical methods
Variables were extracted, explored, and presented as categorical (n [%]) or continuous means (SD) or medians (interquartile range [IQR]). Univariate correlation was assessed with binary logistic regression. Independent factors were sought with binary logistic regression analysis, with each variable within a block being based on a logical clinical progression. The population of more than 150 patients would allow the detection of common variables influencing variance at a level of 2.5%. Thus key, clinically relevant associations should be detected with the analysis. The hierarchical model was built from the variables listed above, with independence confirmed after placement of all independent associations together in a single block. Model regression equations combining factors for the prediction of individual outcomes were developed and parameters estimated, and the area under the curve (AUC) with 95% confidence limits provided. Factors associated with change in HbA1c at 12 months were assessed with general linear regression.
Clinically relevant cutoff values for variables independently associated with outcomes were assessed with receiver operating characteristic (ROC) curves to provide values with the optimal combination of sensitivity and specificity. Individual independent binary predictors were combined to provide a multivariable composite that may be of most clinical value in patient selection and counseling. All analyses were performed with SPSS software (version 19; IBM Corporation, Armonk, NY).
RESULTS
One hundred seventy-four patients were enrolled in the study, but key data were incomplete for 20 subjects and they were excluded from the analysis. The characteristics of the 154 eligible participants are shown in Table 1.
Clinically relevant predictors of diabetes remission (HbA1c ≤6.0%, 42 mmol/mol)
Remission at 12 months was achieved in 107 of the cases (69.5%). Univariate analysis was performed with univariate binary logistic regression. Multiple preoperative factors that were associated with remission at 12 months included younger age, higher BMI, greater waist circumference, a shorter duration of diabetes, not using oral hypoglycemic agents, not using insulin, and higher HOMA, C-peptide, hsCRP, and AST values; however all factors listed in blocks 1–3 were included in the initial phase of the within-block logistic regression. Of clinical importance, baseline HbA1c was not associated with remission in a univariate relationship or when added to the combination models. The mean change in HbA1c at 12 months was 3.3 ± 1.9%, and linear regression analysis found greater reduction in HbA1c between baseline and 12 months to be strongly associated with higher baseline HbA1c (B = −1.03 [95% CI −1.1 to −0.95]; P < 0.001) but largely driven by a statistical floor effect, because only those with quite high values could have large falls in HbA1c (univariate R2 = 0.72). In addition, shorter duration of diabetes (B = 0.05 [0.02–0.95]; P = 0.005) and higher BMI (B = −0.035 [−0.056 to −0.016]; P < 0.001) were also independent predictors of HbA1c reduction (combined R2 = 0.85).
According to the binary logistic regression modeling, the three patient-related variables of shorter diabetes duration, higher fasting C-peptide concentration, and higher BMI were found to be independent predictors of diabetes remission (HbA1c ≤6%), explaining more than a third of variance of remission (Cox and Snell R2 = 0.34) (Table 2). These three continuous variables provide regression parameter estimates, and the likelihood of any individual having remission can be calculated according to the following equation:
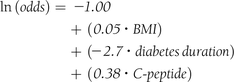
The classification plot indicates correct assignment of 84% of participants to either remission or nonremission. The AUC for the ROC generated is 0.90 (95% CI 0.84–0.95).
A clinically relevant and less mathematically demanding estimate can be derived from cutoff values of each of the 3 continuous variables. Clinically relevant cutoff values were derived from ROC curves by choosing the best combination of sensitivity and specificity for each variable. The selected values, sensitivities, specificities, and AUC, respectively, were 66 and 83%, and 0.74 for BMI >35 kg/m2; 87 and 70%, and 0.79 for duration of diabetes <4 years; and 78, 72, and 75% for C-peptide >2.9 ng/mL. Each patient could be classified as having 0, 1, 2, or 3 of these factors predicting remission. Having ≥2 of the patient-generated factors provided an 82% sensitivity and 87% specificity for remission. Fig. 1 demonstrates the ROC curve with the combined factors and why any combination of ≥2 factors is predictive. According to this scoring system, 60 patients had a score of 0 or 1, and remission was achieved in 19 of these cases (32%), whereas among the 94 patients with a score of ≥2, 88 (94%) were in remission at 12 months. To provide an illustration of the overall effect of each of the predictors, each was divided into quartiles, and the remission rates in each quartile are shown in Fig. 2. The nonlinear effects of duration of diabetes are evident, with poor response in the highest quartile (>5 years) and better response in those with baseline BMI <35.9 kg/m2.
ROC curve for a combination of the three preoperative predictors of diabetes remission (HbA1c ≤6%) with gastric bypass.
ROC curve for a combination of the three preoperative predictors of diabetes remission (HbA1c ≤6%) with gastric bypass.
The three independent preoperative predictors of remission of type 2 diabetes (HbA1c ≤6%) and percentage weight loss at 12 months, showing the quartiles of each variable and the percentage of patients in remission within each quartile. Quartile ranges were as follows: duration of diabetes, <0.5, 0.5–<2, 2.0–<5, and ≥5 years; C-peptide, <2.5, 2.5–<3.4, 3.4–5.3, and ≥5.3 ng/mL; BMI, <30.6, 30.6–<35.9, 35.9–<42, and ≥42 kg/m2; percentage weight loss, <20.7, 20.7–<26.8, 26.8–<33.5, and ≥33.5%.
The three independent preoperative predictors of remission of type 2 diabetes (HbA1c ≤6%) and percentage weight loss at 12 months, showing the quartiles of each variable and the percentage of patients in remission within each quartile. Quartile ranges were as follows: duration of diabetes, <0.5, 0.5–<2, 2.0–<5, and ≥5 years; C-peptide, <2.5, 2.5–<3.4, 3.4–5.3, and ≥5.3 ng/mL; BMI, <30.6, 30.6–<35.9, 35.9–<42, and ≥42 kg/m2; percentage weight loss, <20.7, 20.7–<26.8, 26.8–<33.5, and ≥33.5%.
Predictors of HbA1c >7.0% (53 mmol/mol)
Only 18 (12%) participants had HbA1c >7% at 1 year. The key predictor was duration of diabetes, with 8 years the best cutoff (sensitivity of 78% and specificity of 90% [AUC 0.84]). Fifty-one percent of those not achieving HbA1c ≤7.0% had a history of type 2 diabetes longer than 8 years. C-peptide was also predictive, with 16 of the 18 having a C-peptide level <2.9 ng/mL (sensitivity 89% and specificity 69% [0.84]). These two continuous variables provided a predictive equation for not achieving the HbA1c target of 7%:
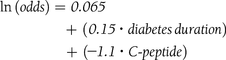
The classification plot would correctly assign 88% of participants to either achieving or not achieving the HbA1c target of ≤ 7%. The AUC for the ROC curve generated is 0.91 (95% CI 0.85–0.95). The key clinical message from combining these predictors is that all patients with diabetes duration <8 years and C-peptide ≥2.9 ng/mL achieved HbA1c ≤7% (AUC for combined factors with cutoffs = 0.91).
Overall, the most important clinical factor was the duration of diabetes, and cutoff values of 4 and 8 years were the best at predicting HbA1c values at 12 months ≤6.0% and ≤7%, respectively. The endogenous insulin production, estimated through C-peptide (2.9 ng/mL), was also an important predictor of achieving target HbA1c levels of ≤6% and ≤7%.
MGB had a significantly higher remission rate than RYGB
MGB had a higher rate of remission (85.5%) compared with RYGB (48.5%; odds ratio [OR] 2.4 [95% CI 1.7–3.4]; P < 0.001). The choice of procedure significantly influenced the targets achieved at all levels. There were, however, major differences in those choosing each procedure after appropriate clinical discussion at baseline (Table 1). Diabetes duration, C-peptide, and MGB independently predicted HbA1c ≤6.0%, and diabetes duration and MGB independently predicted HbA1c >7.0%. No other factors were predictive when the type of surgery was modeled. After adjustment for other predictors of remission, the OR for MGB was 3.9 (1.5–9.8). The additional effect of MGB may have been associated with additional weight loss (see below).
What was the influence of weight loss?
Initial mean BMI was 37.2 (SD 8.9), median 35.9 (IQR 30.6–42.0, range 23–94 kg/m2). Percentage weight loss was therefore used to examine the influence of weight loss as actual weight loss and percentage of excess weight loss are distorted by the large BMI range. The duration of diabetes, baseline C-peptide and percentage weight loss strongly and independently influenced remission at 1 year (Column 5, Table 2). The predictive equation with parameter estimates for and individual for remission is:
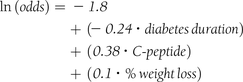
The AUC for the ROC produced was 9.0 (0.84–0.96). MGB produced considerably greater percentage weight loss (29.5 ± 8.5%) compared with RYGB (24.2 ± 10.2%; P = 0.001) in the first 12 months after surgery. After controlling for weight loss, duration of diabetes, and C-peptide, the type of surgery was no longer an influence on remission. Remission rates for quartiles of weight loss and preoperative predictive factors are shown in Fig. 2.
The following ROC curve–derived cutoff values for likelihood of remission were each assigned a score of 1: duration of diabetes <4 years, percentage weight loss at 1 year >25% , and C-peptide ≥2.9 ng/mL at baseline. A cumulative score of 2 or 3 was associated with a remission rate of 92%, whereas a score of 0 or 1 was associated with a remission rate of only 27%.
Greater percentage weight loss, shorter duration of diabetes, and higher baseline HbA1c were all independent predictors of change in HbA1c at 12 months (combined R2 = 0.86).
Are predictive factors related to one another?
Although the predictive factors identified in this study independently explain statistical variance for remission, they are clinically associated. Increasing BMI is associated with elevated fasting C-peptide concentrations (r = 0.56; P < 0.001), probably as a result of increased insulin resistance, and duration of type 2 diabetes is associated with reduced concentrations (r = −0.41; P < 0.001), which is related to reduced β-cell function. Despite these important clinical associations, parameter estimates did not vary in any major way between univariate and multivariate analyses, and parameters were assessed for clinical and biological plausibility. Importantly, none changed from positive to negative or vice versa, and none had a correlation coefficient greater than 0.7.
There was no mortality in this series, and there were only two major complications (1.3%); both were upper gastrointestinal bleeding, one after RYGB and the other after MGB. Another 17 minor complication (11.0%), 8 after RYGB and 9 after MGB, were documented in the series.
CONCLUSIONS
This analysis has identified three independent and clinically important preoperative factors that are related to outcomes of bariatric-metabolic surgery in an ethnic Chinese population after gastric bypass. Longer duration of diabetes, lower C-peptide level, and lower BMI reduce the likelihood of response to surgery. These factors, although independent statistically, are also interrelated. High C-peptide levels are a response to insulin resistance and represent increased endogenous insulin production. The association of higher C-peptide levels with increasing BMI (13) and that of lower levels with increasing duration of type 2 diabetes were confirmed in this study. Further, very low C-peptide levels are efficient at identifying type 1 diabetes and latent autoimmune diabetes in adults (14). The combination of factors indicates that responders are likely to have increased fatness, to be more insulin resistant, and to have good or adequate residual β-cell function. Lower baseline BMI and longer duration of diabetes also predicted a reduced fall in baseline HbA1c. In this study, these factors not only predicted remission but were useful in identifying those unlikely to have HbA1c ≤7% after surgery and thus may help to identify preoperatively those patients who will have a poor result after surgery. Cutoff values and regression equations provide guidance to the clinician regarding patient selection and will help in counseling patients.
MGB produced better levels of diabetes remission at 12 months, but this may be related to patient choice in procedure selection and greater weight loss. Weight loss after gastric bypass is very important to glycemic outcomes, but this does not exclude the putative glycemic effects of gastric bypass not related to weight loss (15). The importance of increasing BMI, increasing insulin requirements, and weight loss to overall outcomes cannot be ignored, however, and indicates that this is indeed bariatric-metabolic surgery, and we should be cautious about extrapolation of the benefits of surgery to diabetic patients who have lower BMI and lower insulin requirements.
Several recent studies have alerted us to the danger of ignoring fatness and weight loss when considering bariatric-metabolic surgery. Scopinaro et al. (16,17) have shown bariatric-metabolic surgery to be less effective in individuals with lower BMI, thereby indicating that the surgical results are not independent of initial BMI or weight loss. A large Korean (18) study examined the effect of gastric cancer surgery on remission of type 2 diabetes. That study, which comprised 403 patients with diabetes and a mean BMI of 24.7, found remission to be related to both increasing baseline BMI and postsurgical weight loss. The extent of gastric resection and type of reconstruction did not influence outcomes. A recent review of bariatric-metabolic surgery in the BMI range <35 kg/m2 included multiple cohorts with a mean BMI <30 kg/m2. In these studies, HbA1c changes were variable, they did not reach the glycemic control demonstrated in patients with initially higher BMI, and change in mean HbA1c was related to the extent of weight loss. Some of these cohorts with little weight loss after a bariatric procedure demonstrated no significant change in HbA1c (19). These findings are confirmed in the current study, in which we have identified the characteristics of those unlikely to achieve HbA1c ≤7% and those unlikely to achieve a substantial fall in HbA1c. Increasing age is an important consideration for patient selection as well, because the risks of both surgery and weight loss increase with age and benefits are attenuated, especially if diabetes is long-standing (20,21).
There are strengths and weaknesses in this analysis. Most importantly, these results apply to a single ethnic group, and all patients had gastric bypass surgery. This homogeneity allowed a clear definition of the key predictors of outcome to emerge, and although these are consistent with data from those of European ethnicity, care is needed when extrapolating both to other ethnic groups and to other surgical procedures. It is also important to confirm these associations in another cohort of ethnic Chinese patients with type 2 diabetes. Some caution is required in assessing C-peptide cutoff values, because individuals with low levels (<0.9 ng/mL) were excluded from the analysis, and assay results can vary with the methods used and between laboratories (22). In addition, although the principles may apply to other ethnicities and procedures, the exact predictive equations and cutoff values are likely to vary with the characteristics of the cohort.
In conclusion, patients with type 2 diabetes of Chinese ethnicity from Taiwan have a glycemic response to gastric bypass surgery that is related to BMI, duration of diabetes, fasting C-peptide (an indicator of insulin secretion and residual β-cell function), and weight loss. Caution is required in extrapolating the benefits of surgery to diabetic patients who have lower BMI. Although they may provide useful clinical guidance, these factors should be confirmed in separate cohorts, with other ethnicities and bariatric procedures.
Acknowledgments
This study was supported by a research grant from Min-Sheng General Hospital, Taiwan. J.B.D., G.W.L., N.E.S., and E.A.L. receive research support from the National Health and Medical Research Council of Australia. The Baker IDI Heart and Diabetes Institute is supported in part by the Victorian Government's OIS Program. J.B.D. is a consultant for Allergan Inc. and Metagenics Inc. (Bariatric Advantage). He is also on the medical advisory board for Optifast, Nestle Australia. The laboratory of J.B.D., G.W.L., N.E.S., and E.A.L. at Baker IDI Heart and Diabetes Institute currently receives research funding from Medtronic Inc. (formally Ardian Inc), Abbott (formerly Solvay) Pharmaceuticals, Allergan Inc., Servier, and Scientific Intake. The funding organizations played no role in the design, analysis, or interpretation of data described here, nor in preparation, review, or approval of the manuscript. No other potential conflicts of interest relevant to this article were reported.
J.B.D. developed the aims and hypotheses for the study with W.J.L., performed the data analysis, and wrote the manuscript. L.-M.C. and K.C. (endocrinologists) and S.-C.C. (diabetes educator) provided postsurgical diabetes care management, collected the data, contributed to the interpretation of findings, and reviewed and edited the manuscript. G.W.L. and N.E.S. contributed significantly to discussion of the findings and reviewed and edited the manuscript. E.A.L. reviewed the manuscript and contributed to discussion. W.J.L. performed the bariatric procedures, collected the data for the study, provided background information, significantly contributed to the interpretation of findings, and reviewed the manuscript. W.J.L. is the guarantor of this work and, as such, had full access to all the data in the study and takes responsibility for the integrity of the data and the accuracy of the data analysis.
Parts of this study were presented in oral form at the 72nd Scientific Sessions of the American Diabetes Association, Philadelphia, Pennsylvania, 8–12 June 2012; and as an oral presentation at the International Federation for the Surgery of Obesity and Metabolic Disorders (IFSO) Annual Meeting, New Delhi, India, 11–15 September 2012.
The authors thank Michael Bailey for his statistical advice regarding the methodology used in this analysis.